How a Hawk Scholar is Bringing Baseball Analytics to Life with AI
Nicolas Zywalewski, BS ’26, and Abolfazl Saghafi, PhD, are using deep learning to recreate pro-level baseball stats at a fraction of the cost.
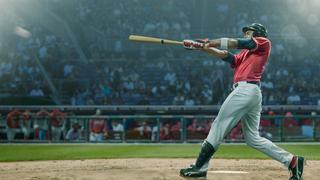
In Major League Baseball, there’s no shortage of data and analytics being put into play. From tracking player performance to measuring every swing of the bat, teams rely on sophisticated systems, which use thousands of cameras and advanced AI to deliver real-time metrics. But what if you could recreate some of these analytics with a fraction of the budget and equipment?
That’s exactly what Nicolas Zywalewski, BS '26, a data science and mathematics double major at Saint Joseph’s University, set out to do. Partnering with Abolfazl Saghafi, PhD, associate professor of mathematics, Zywalewski took on the challenge of building a deep learning model to calculate exit velocities of baseballs — all using just a laptop and video footage available online.
Zywalewski has always been a self-described “math guy” and has played baseball since a young age. It was those two interests that propelled him and Saghafi to work on the ambitious project through the University’s Summer Scholars Program.
“I’ve played baseball my whole life, and it’s a very math-heavy sport,” he says, recognizing the rapid growth of analytics in the game. “I’ve been able to combine two of my passions with this research.”
The project, which focuses on using deep learning neural networks, specifically YOLO (You Only Look Once) models, is designed to track baseballs and bats in video footage to determine key metrics like exit velocity.
Deep learning neural networks, as Saghafi explains, are a subset of AI designed to make decisions much like a human would, but at faster speeds and with greater accuracy.
"These models learn by examples; you provide them with thousands of images or video frames where objects of interest are boxed, allowing them to recognize boxed objects — in this case, baseballs and bats," says Saghafi.
Through an optimization process, the model learns to distinguish the ball and the bat in images and video frames. Then that speed of the ball being hit can be calculated using a few consecutive images.
Once we trained the model, we were able to calculate exit velocities within just a few miles per hour of the actual data provided by the MLB.
Nicolas Zywalewski, BS '26
The project began with Zywalewski combing through MLB footage, pulling 28 videos with different angles and extracting key frames to build a dataset of images. He then annotated these images, boxing the location of the baseballs and bats, which were fed into the neural network for training.
“Once we trained the model, we were able to calculate exit velocities within just a few miles per hour of the actual data provided by the MLB,” Zywalewski says. “By the end of summer, I was like, ‘Wow we actually did pretty good, despite just finding the videos online and not having the millions of data points that the MLB has.'”
While currently focused on baseball, this model has the potential to extend to other sports. Saghafi’s Data Science for Sports class will use similar technology to analyze soccer and tennis matches.
“It makes it very interesting for students to see the applications of mathematics in something they love, like sports," says Saghafi. “They learn the math and statistics behind the game.”
Zywalewski’s passion for sports analytics also led him to start the University’s Sports Analytics Club, a space where like-minded students can explore the growing field together. The club recently held its first meeting, discussing analytics for the NFL season and baseball awards predictions.
Having a space to explore his interests, as well as having close relationships with professors like Saghafi, were key reasons for Zywalewski choosing to attend SJU.
"Whether it is aiding in finding an internship or telling me about courses that will be running in future semesters, [professors] are constantly showing their support. That close-knit community has been even better than I expected,” says Zywalewski.